Differentiable Programming With Swift: A Comprehensive Guide

Differentiable programming is a powerful technique that allows you to write code that can automatically compute gradients. This makes it possible to train machine learning models and optimize complex functions with ease.
In this article, we'll explore the basics of differentiable programming with Swift and show you how to use it to solve a variety of real-world problems.
Differentiable programming is a technique for writing code that can be differentiated automatically. This means that you can write code to compute the gradient of a function with respect to its inputs, without having to write the gradient calculation yourself.
4.4 out of 5
Language | : | English |
File size | : | 8427 KB |
Text-to-Speech | : | Enabled |
Screen Reader | : | Supported |
Enhanced typesetting | : | Enabled |
Print length | : | 306 pages |
This is a very powerful capability, as it allows you to train machine learning models and optimize complex functions with ease.
Differentiable programming works by using a technique called automatic differentiation. Automatic differentiation is a process of automatically computing the gradient of a function with respect to its inputs.
There are a number of different automatic differentiation algorithms, but the most common one is called the backpropagation algorithm. The backpropagation algorithm works by propagating the gradients of a function through its computation graph.
The computation graph is a directed graph that represents the computation of a function. The nodes of the graph represent the operations that are performed in the computation, and the edges of the graph represent the data that flows between the operations.
The backpropagation algorithm starts by computing the gradient of the output of the function with respect to its inputs. It then propagates these gradients through the computation graph, using the chain rule to compute the gradients of the intermediate operations.
Once the backpropagation algorithm has finished, you have the gradients of all of the operations in the computation graph. This information can be used to train machine learning models and optimize complex functions.
Differentiable programming offers a number of benefits over traditional programming techniques. These benefits include:
- Ease of use: Differentiable programming makes it easy to train machine learning models and optimize complex functions. You don't have to write the gradient calculations yourself, which can save you a lot of time and effort.
- Efficiency: Differentiable programming is very efficient. The backpropagation algorithm can be implemented using a variety of techniques that make it very fast.
- Flexibility: Differentiable programming can be used to solve a wide variety of problems. It is not limited to machine learning or optimization.
Differentiable programming has a wide range of applications, including:
- Machine learning: Differentiable programming is used to train machine learning models. This includes supervised learning, unsupervised learning, and reinforcement learning.
- Optimization: Differentiable programming can be used to optimize complex functions. This includes finding the minimum or maximum of a function, or finding the optimal solution to a constrained optimization problem.
- Computer graphics: Differentiable programming can be used to create realistic computer graphics. This includes rendering images, animating characters, and simulating physical phenomena.
To get started with differentiable programming in Swift, you will need to install the Swift for TensorFlow library. Swift for TensorFlow is a library that provides a high-level API for differentiable programming in Swift.
Once you have installed Swift for TensorFlow, you can start writing differentiable code. The following code shows how to compute the gradient of a simple function:
swift import TensorFlow
func f(x: Tensor) -> Tensor { return x * x }
let x = Tensor(1.0) let gradient = gradient(at: x){f($0) }
print(gradient) // Output: [2.0]
In this example, we define a simple function f(x) = x^2. We then compute the gradient of f(x) with respect to x using the gradient() function. The output of the gradient() function is a tensor that contains the gradient of f(x) at the point x.
Differentiable programming is a powerful technique that allows you to write code that can automatically compute gradients. This makes it possible to train machine learning models and optimize complex functions with ease.
In this article, we explored the basics of differentiable programming with Swift and showed you how to use it to solve a variety of real-world problems.
We encourage you to explore the resources that we have provided and to experiment with differentiable programming yourself. We believe that differentiable programming has the potential to revolutionize the way that we develop software.
4.4 out of 5
Language | : | English |
File size | : | 8427 KB |
Text-to-Speech | : | Enabled |
Screen Reader | : | Supported |
Enhanced typesetting | : | Enabled |
Print length | : | 306 pages |
Do you want to contribute by writing guest posts on this blog?
Please contact us and send us a resume of previous articles that you have written.
Book
Novel
Page
Text
Story
Magazine
Paragraph
Bookmark
Bibliography
Foreword
Synopsis
Footnote
Manuscript
Scroll
Codex
Tome
Bestseller
Classics
Narrative
Biography
Encyclopedia
Thesaurus
Resolution
Catalog
Card Catalog
Stacks
Archives
Periodicals
Study
Research
Scholarly
Journals
Reading Room
Rare Books
Interlibrary
Literacy
Study Group
Thesis
Storytelling
Reading List
Tom Townsend
Don Charlwood
Madeleine Bourdouxhe
Jay Althouse
Melanie Wylutzki
Richard Platt
Patrick Anderson
Susan Lockwood
Jenny Tomlin
Andrew Davies
J M Synge
Sara Novic
Yong Chen
Andrea Tantaros
Tim Zak
Melinda Metz
Pamela Horn
Soul Sparkle
Jamie Smart
Andrew Marshall Wayment
Light bulbAdvertise smarter! Our strategic ad space ensures maximum exposure. Reserve your spot today!
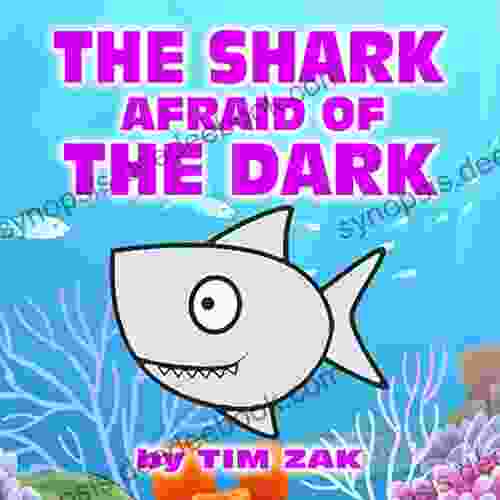

- Raymond ChandlerFollow ·9.8k
- Gabriel MistralFollow ·4.1k
- Cruz SimmonsFollow ·3.3k
- Robert BrowningFollow ·8.5k
- Darren BlairFollow ·3.7k
- Louis HayesFollow ·3.3k
- Glen PowellFollow ·18.2k
- Banana YoshimotoFollow ·14.8k
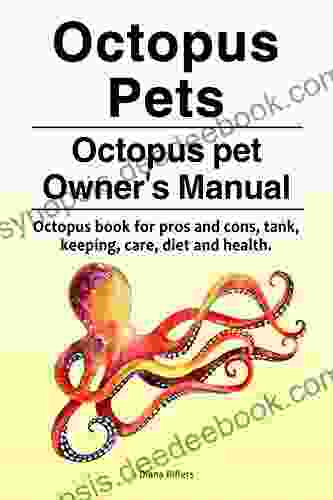

Octopus as Pets: A Comprehensive Guide to Care, Costs,...
Octopuses are...
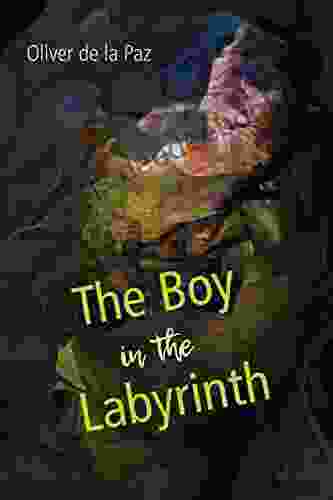

Akron, Ohio: A City of Poems
Akron, Ohio is a city with...
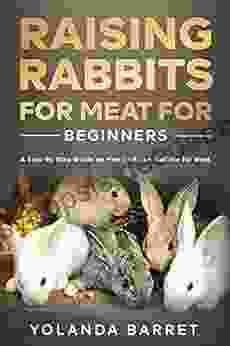

A Comprehensive Guide to Raising Rabbits for Meat
Rabbit meat is a nutritious and sustainable...
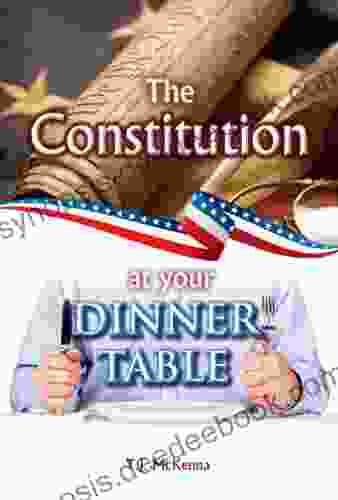

The Constitution at Your Dinner Table: How the Founding...
The United States...
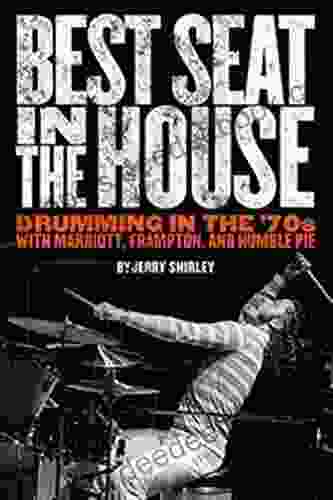

Drumming in the 70s with Marriott, Frampton, and Humble...
The 1970s was a...
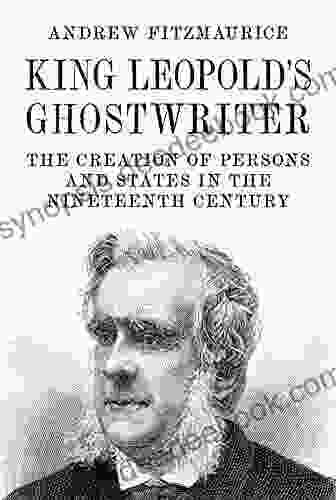

The Creation of Persons and States in the Nineteenth...
The nineteenth century...
4.4 out of 5
Language | : | English |
File size | : | 8427 KB |
Text-to-Speech | : | Enabled |
Screen Reader | : | Supported |
Enhanced typesetting | : | Enabled |
Print length | : | 306 pages |